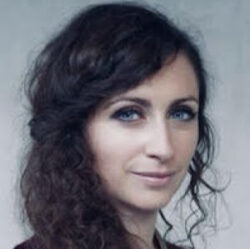
TILOS Seminar: Single location regression and attention-based models
2pm PDT | Thursday, March 27, 2025
Claire Boyer, Université Paris-Saclay
Abstract: Attention-based models, such as Transformer, excel across various tasks but lack a comprehensive theoretical understanding, especially regarding token-wise sparsity and internal linear representations. To address this gap, we introduce the single-location regression task, where only one token in a sequence determines the output, and its position is a latent random variable, retrievable via a linear projection of the input. To solve this task, we propose a dedicated predictor, which turns out to be a simplified version of a non-linear self-attention layer. We study its theoretical properties, by showing its asymptotic Bayes optimality and analyzing its training dynamics. In particular, despite the non-convex nature of the problem, the predictor effectively learns the underlying structure. This work highlights the capacity of attention mechanisms to handle sparse token information and internal linear structures.